TODS:自动化时间序列异常检测系统
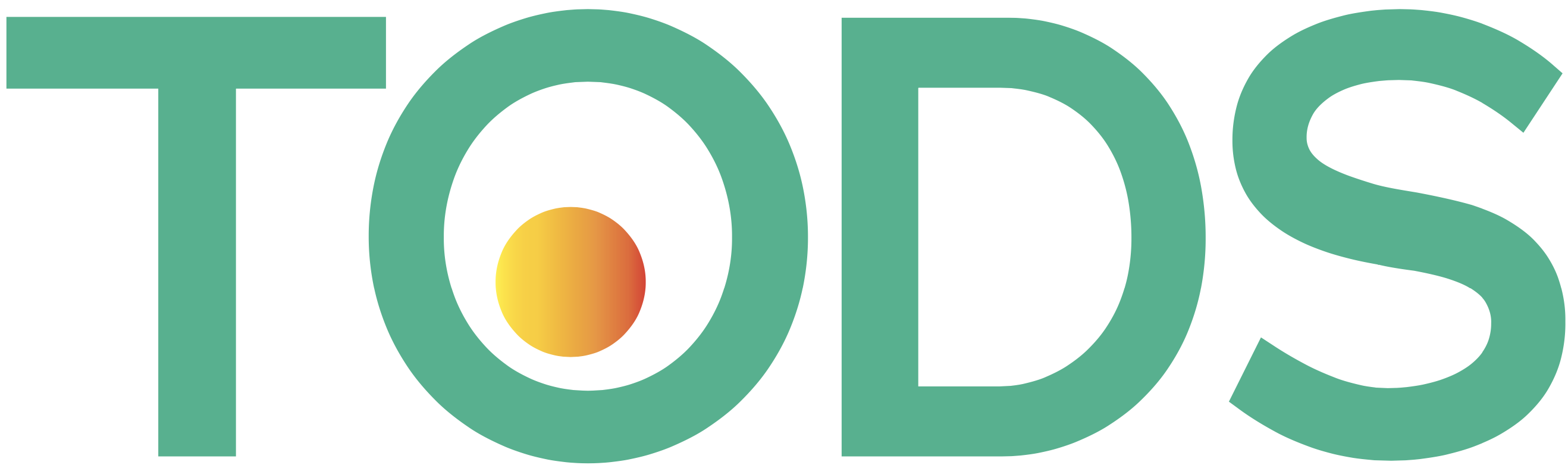
TODS是一个用于多变量时间序列数据异常检测的全栈自动化机器学习系统。TODS提供了构建基于机器学习的异常检测系统的全面模块,包括:数据处理、时间序列处理、特征分析(提取)、检测算法和强化模块。这些模块提供的功能包括通用数据预处理、时间序列数据平滑/转换、从时间/频率域提取特征、各种检测算法,以及引入人类专业知识来校准系统。TODS可以执行时间序列数据上的三种常见异常检测场景:点级检测(时间点作为异常)、模式级检测(子序列作为异常)和系统级检测(时间序列集合作为异常),并提供了广泛的相应算法。该软件包由Rice大学DATA实验室开发。
TODS的特点包括:
-
全栈机器学习系统,支持从预处理、特征提取、检测算法到人机交互界面的全面组件。
-
广泛的算法,包括PyOD支持的所有点级检测算法,最先进的模式级(集体)检测算法如DeepLog、Telemanon,以及用于执行系统级检测的各种集成算法。
-
自动化机器学习旨在提供无需知识的处理过程,通过自动搜索所有现有模块的最佳组合,基于给定数据构建最优管道。
示例和教程
资源
- API文档:http://tods-doc.github.io
- 论文:https://arxiv.org/abs/2009.09822
- 相关项目:AutoVideo:自动视频动作识别系统
- :扬声器: 想了解更多关于数据流程搜索的信息吗?请查看我们的数据中心AI调查和数据中心AI资源!
引用本工作:
如果您觉得本工作有用,可以引用:
@article{Lai_Zha_Wang_Xu_Zhao_Kumar_Chen_Zumkhawaka_Wan_Martinez_Hu_2021,
title={TODS: An Automated Time Series Outlier Detection System},
volume={35},
number={18},
journal={Proceedings of the AAAI Conference on Artificial Intelligence},
author={Lai, Kwei-Herng and Zha, Daochen and Wang, Guanchu and Xu, Junjie and Zhao, Yue and Kumar, Devesh and Chen, Yile and Zumkhawaka, Purav and Wan, Minyang and Martinez, Diego and Hu, Xia},
year={2021}, month={May},
pages={16060-16062}
}
安装
本软件包适用于**Python 3.7+**和pip 19+。您需要在系统上安装以下软件包(适用于Debian/Ubuntu):
sudo apt-get install libssl-dev libcurl4-openssl-dev libyaml-dev build-essential libopenblas-dev libcap-dev ffmpeg
克隆仓库(如果您在中国且Github访问速度较慢,可以使用Gitee上的镜像):
git clone https://github.com/datamllab/tods.git
使用pip
在本地安装:
cd tods
pip install -e .
示例
示例可在/examples中找到。对于基本用法,您可以在给定的数据集上评估一个流程。这里,我们提供了一个示例,加载我们的默认流程并在yahoo数据集的一个子集上评估它。
import pandas as pd
from tods import schemas as schemas_utils
from tods import generate_dataset, evaluate_pipeline
table_path = 'datasets/anomaly/raw_data/yahoo_sub_5.csv'
target_index = 6 # 目标列的索引
metric = 'F1_MACRO' # 标签0和1的F1值
# 读取数据并生成数据集
df = pd.read_csv(table_path)
dataset = generate_dataset(df, target_index)
# 加载默认流程
pipeline = schemas_utils.load_default_pipeline()
# 运行流程
pipeline_result = evaluate_pipeline(dataset, pipeline, metric)
print(pipeline_result)
我们还提供AutoML支持,帮助您自动为您的数据找到一个好的流程。
import pandas as pd
from axolotl.backend.simple import SimpleRunner
from tods import generate_dataset, generate_problem
from tods.searcher import BruteForceSearch
# 一些信息
table_path = 'datasets/yahoo_sub_5.csv'
target_index = 6 # 目标列的索引
time_limit = 30 # 您想搜索的秒数
metric = 'F1_MACRO' # 标签0和1的F1值
# 读取数据并生成数据集和问题
df = pd.read_csv(table_path)
dataset = generate_dataset(df, target_index=target_index)
problem_description = generate_problem(dataset, metric)
# 启动后端
backend = SimpleRunner(random_seed=0)
# 启动搜索算法
search = BruteForceSearch(problem_description=problem_description,
backend=backend)
# 寻找最佳流水线
best_runtime, best_pipeline_result = search.search_fit(input_data=[dataset], time_limit=time_limit)
best_pipeline = best_runtime.pipeline
best_output = best_pipeline_result.output
# 评估最佳流水线
best_scores = search.evaluate(best_pipeline).scores
致谢
我们衷心感谢美国国防高级研究计划局(DARPA)的数据驱动模型发现(D3M)项目