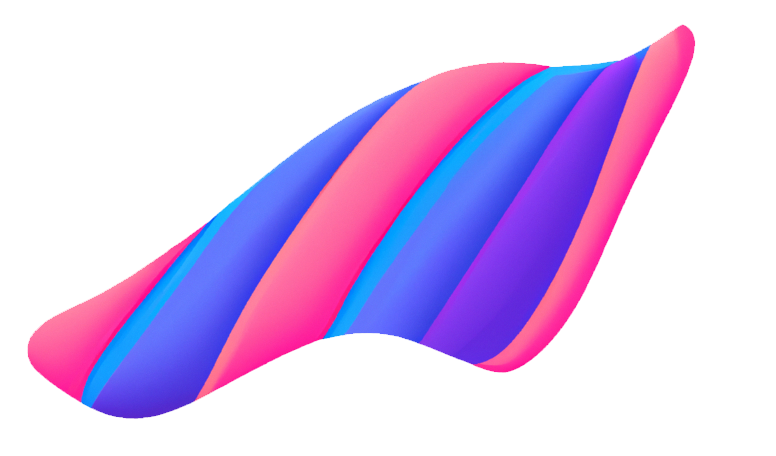
dasp
PyTorch中的可微分音频信号处理器
包括混响、失真、动态范围处理、均衡、立体声处理。
支持虚拟模拟建模、盲参数估计、自动化DSP和风格迁移。
批处理可在CPU和GPU加速器上运行,实现快速训练并减少瓶颈。
开源且可在Apache 2.0许可下免费用于学术和商业应用。
安装
pip install dasp-pytorch
或者,进行本地安装。
git clone https://github.com/csteinmetz1/dasp-pytorch
cd dasp-pytorch
pip install -e .
示例
dasp-pytorch
是一个Python库,用于使用PyTorch构建可微分音频信号处理器。
这些可微分处理器可以单独使用或在神经网络的计算图中使用。
我们为所有处理器提供纯函数接口,以便于使用并在项目间移植。
除非另有说明,所有效果函数都期望输入和输出形状为(batch_size, num_channels, num_samples)
的3维张量。
在计算图中使用效果就像调用以输入张量为参数的函数一样简单。
快速入门
这里有一个最小示例,演示如何使用梯度下降反向工程简单失真效果的驱动值。
import torch
import torchaudio
import dasp_pytorch
# 加载音频
x, sr = torchaudio.load("audio/short_riff.wav")
# 创建批次维度
# (batch_size, n_channels, n_samples)
x = x.unsqueeze(0)
# 应用16 dB驱动的失真
drive = torch.tensor([16.0])
y = dasp_pytorch.functional.distortion(x, sr, drive)
# 创建一个要优化的参数
drive_hat = torch.nn.Parameter(torch.tensor(0.0))
optimizer = torch.optim.Adam([drive_hat], lr=0.01)
# 优化参数
n_iters = 2500
for n in range(n_iters):
# 用估计的参数应用失真
y_hat = dasp_pytorch.functional.distortion(x, sr, drive_hat)
# 计算估计值与目标之间的距离
loss = torch.nn.functional.mse_loss(y_hat, y)
# 优化
optimizer.zero_grad()
loss.backward()
optimizer.step()
print(
f"步骤: {n+1}/{n_iters}, 损失: {loss.item():.3e}, 驱动: {drive_hat.item():.3f}\r"
)
对于剩余的示例,我们将使用GuitarSet数据集。 你可以使用以下命令下载数据:
mkdir data
wget https://zenodo.org/records/3371780/files/audio_mono-mic.zip
unzip audio_mono-mic.zip
rm audio_mono-mic.zip
更多示例
音频处理器
音频处理器 | 函数接口 |
---|---|
增益 | gain() |
失真 | distortion() |
参数均衡器 | parametric_eq() |
动态范围压缩器 | compressor() |
动态范围扩展器 | expander() |
混响 | noise_shaped_reverberation() |
立体声扩展器 | stereo_widener() |
立体声声像 | stereo_panner() |
立体声总线 | stereo_bus() |
引用
如果您使用了这个库,请考虑引用以下论文:
可微分参数均衡器和动态范围压缩器
@article{steinmetz2022style,
title={Style transfer of audio effects with differentiable signal processing},
author={Steinmetz, Christian J and Bryan, Nicholas J and Reiss, Joshua D},
journal={arXiv preprint arXiv:2207.08759},
year={2022}
}
具有频带噪声整形的可微分人工混响
@inproceedings{steinmetz2021filtered,
title={Filtered noise shaping for time domain room impulse
response estimation from reverberant speech},
author={Steinmetz, Christian J and Ithapu, Vamsi Krishna and Calamia, Paul},
booktitle={WASPAA},
year={2021},
organization={IEEE}
}
可微分IIR滤波器
@inproceedings{nercessian2020neural,
title={Neural parametric equalizer matching using differentiable biquads},
author={Nercessian, Shahan},
booktitle={DAFx},
year={2020}
}
@inproceedings{colonel2022direct,
title={Direct design of biquad filter cascades with deep learning
by sampling random polynomials},
author={Colonel, Joseph T and Steinmetz, Christian J and
Michelen, Marcus and Reiss, Joshua D},
booktitle={ICASSP},
year={2022},
organization={IEEE}
致谢
由EPSRC UKRI人工智能与音乐博士培训中心(EP/S022694/1)支持。